
Chemistry computer algorithm does more with less experimental data to reveal the optimal way to make green materials.
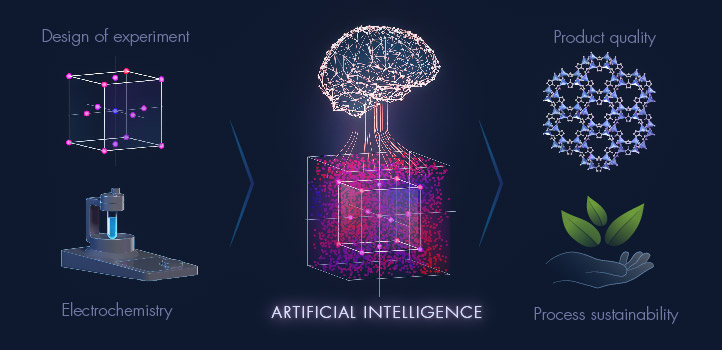
Machine learning and design of experiments can be combined to identify the most sustainable method for developing advanced materials.
© 2020 KAUST; Xavier Pita
Machine learning could teach us how to make the manufacturing of materials cleaner and more sustainable by taking a holistic view to identify the greenest production method, suggest KAUST researchers.
The quest for sustainability means that scientists worldwide are developing advanced materials to help address issues, including carbon capture, water desalination and energy storage, says Rifan Hardian, a postdoc in Gyorgy Szekely’s lab. “Although these materials show promising performance, the materials themselves are often produced in unsustainable ways—using harsh conditions, toxic solvents and energy-intensive processes that generate excessive waste—potentially creating more environmental problems than they solve,” Hardian says.
In collaboration with Xiangliang Zhang and his team, Szekely and Hardian have been investigating a more sustainable approach to materials development, called design of experiments (DoE). “Unlike conventional approaches to materials optimization, which vary one factor at a time, DoE is a systematic approach that allows multiple factors to be varied simultaneously,” Hardian says.
DoE theoretically allows variables—such as reactant and solvent choice, reaction time and reaction temperature—to be optimized all at once. The procedure cuts the number of experiments conducted and also potentially identifies the greenest possible way to make a material. However, it is challenging to optimize each variable to identify the best reaction protocol from such sparse experimental data. “This is where machine learning comes in,” Hardian says.
Machine learning is a form of artificial intelligence that can learn patterns from a limited number of data points to fill in the blanks in the data. “This way, one can view the entire experimental space and pick the one reaction condition that best fits the desired results,” Hardian says.
The team combined DoE and machine learning to identify a sustainable method for making a popular metal organic framework (MOF) material called ZIF-8. “ZIF-8 has great potential in applications, such as gas separation, catalysis, heavy metal removal and environmental remediation,” Hardian says. The team optimized 10 variables in the electrochemical synthesis of ZIF-8, identifying a high-yielding process that used water as a solvent and generated minimal waste. “Thanks to machine learning, we developed a holistic view of the variables’ interactions and identified many unexpected correlations that could have been missed if we had followed a conventional approach,” Hardian says.
The next milestone will be to apply DoE and machine learning to large-scale materials production, Szekely says. “Ultimately, our aim is to turn the futuristic vision of an autonomous laboratory system, which can continuously run and self-optimize reaction conditions, into a reality,” he says.
KAUST DISCOVERY
Related Publications
- Artificial intelligence: the silver bullet for sustainable materials development
R. Hardian, Z. Liang, X. Zhang, G. Szekely
Green Chem.,22, 7521-7528, (2020)